Computational photography is the use of clever light-gathering tricks and sophisticated algorithms to extract more information from the visual environment than traditional cameras can.
The first commercial application of computational photography is the so-called light-field camera, which can measure not only the intensity of incoming light but also its angle. That information can be used to produce multiperspective 3-D images, or to refocus a shot even after it’s been captured.
Existing light-field cameras, however, trade a good deal of resolution for that extra angle information: A camera with a 20-megapixel sensor, for instance, will yield a refocused image of only one megapixel.
Researchers in the Camera Culture Group at MIT’s Media Lab aim to change that with a system they’re calling Focii. At this summer’s Siggraph — the major computer graphics conference — they’ll present a paper demonstrating that Focii can produce a full, 20-megapixel multiperspective 3-D image from a single exposure of a 20-megapixel sensor.
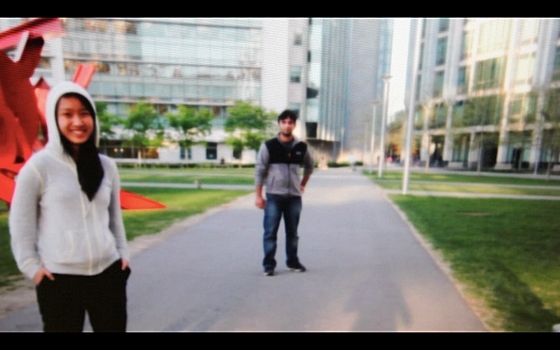
Moreover, while a commercial light-field camera is a $400 piece of hardware, Focii relies only on a small rectangle of plastic film, printed with a unique checkerboard pattern, that can be inserted beneath the lens of an ordinary digital single-lens-reflex camera. Software does the rest.
Gordon Wetzstein, a postdoc at the Media Lab and one of the paper’s co-authors, says that the new work complements the Camera Culture Group’s ongoing research on glasses-free 3-D displays. “Generating live-action content for these types of displays is very difficult,” Wetzstein says. “The future vision would be to have a completely integrated pipeline from live-action shooting to editing to display. We’re developing core technologies for that pipeline.”
In 2007, Ramesh Raskar, the NEC Career Development Associate Professor of Media Arts and Sciences and head of the Camera Culture Group, and colleagues at Mitsubishi Electric Research showed that a plastic film with a pattern printed on it — a “mask” — and some algorithmic wizardry could produce a light-field camera whose resolution matched that of cameras that used arrays of tiny lenses, the approach adopted in today’s commercial devices. “It has taken almost six years now to show that we can actually do significantly better in resolution, not just equal,” Raskar says.
Split atoms
Focii represents a light field as a grid of square patches; each patch, in turn, consists of a five-by-five grid of blocks. Each block represents a different perspective on a 121-pixel patch of the light field, so Focii captures 25 perspectives in all. (A conventional 3-D system, such as those used to produce 3-D movies, captures only two perspectives; with multiperspective systems, a change in viewing angle reveals new features of an object, as it does in real life.)
The key to the system is a novel way to represent the grid of patches corresponding to any given light field. In particular, Focii describes each patch as the weighted sum of a number of reference patches — or “atoms” — stored in a dictionary of roughly 5,000 patches. So instead of describing the upper left corner of a light field by specifying the individual values of all 121 pixels in each of 25 blocks, Focii simply describes it as some weighted combination of, say, atoms 796, 23 and 4,231.
According to Kshitij Marwah, a graduate student in the Camera Culture Group and lead author on the new paper, the best way to understand the dictionary of atoms is through the analogy of the Fourier transform, a widely used technique for decomposing a signal into its constituent frequencies.
In fact, visual images can be — and frequently are — interpreted as signals and represented as sums of frequencies. In such cases, the different frequencies can also be represented as atoms in a dictionary. Each atom simply consists of alternating bars of light and dark, with the distance between the bars representing frequency.
The atoms in the Camera Culture Group researchers’ dictionary are similar but much more complex. Each atom is itself a five-by-five grid of 121-pixel blocks. Each block consists of arbitrary-seeming combinations of color: The blocks in one atom might all be green in the upper left corner and red in the lower right, with lines at slightly different angles separating the regions of color; the blocks of another atom might all feature slightly different-size blobs of yellow invading a region of blue.
Behind the mask
In building their dictionary of atoms, the Camera Culture Group researchers — Marwah, Wetzstein, Raskar, and Yosuke Bando, a visiting scientist at the Media Lab — had two tools at their disposal that Joseph Fourier, working in the late 18th century, lacked: computers, and lots of real-world examples of light fields.
To build their dictionary, they turned a computer loose to try out lots of different combinations of colored blobs and determine which, empirically, enabled the most efficient representation of actual light fields.
Once the dictionary was built, however, they still had to calculate the optimal design of the mask they use to record light-field information — the patterned plastic film that they slip beneath the camera lens. Bando explains the principle behind mask design using, again, the analogy of Fourier transform.
“If a mask has a particular frequency in the vertical direction” — say, a regular pattern of light and dark bars — “you only capture that frequency component of the image,” Bando says. “So you have no way of recovering the other frequencies. If you use frequency domain reconstruction, the mask should contain every frequency in a systematic manner.”
“Think of atoms as the new frequency,” Marwah says. “In our case, we need a mask pattern that can effectively cover as many atoms as possible.”
“It’s cool work,” says Kari Pulli, senior director of research at graphics-chip company Nvidia. “Especially the idea that you can take video at fairly high resolution — that’s kind of exciting.”
Pulli points out, however, that assembling an image from the information captured by the mask is currently computationally intensive. Moreover, he says, the examples of light fields used to assemble the dictionary may have omitted some types of features common in the real world. “There’s still work to be done for this to be actually something that consumers would embrace,” Pulli says.