In today’s computers, moving data to and from main memory consumes so much time and energy that microprocessors have their own small, high-speed memory banks, known as “caches,” which store frequently used data. Traditionally, managing the caches has required fairly simple algorithms that can be hard-wired into the chips.
In the 21st century, however, in order to meet consumers’ expectations for steadily increasing computational power, chipmakers have had to begin equipping their chips with more and more cores, or processing units. And as cores proliferate, cache management becomes much more difficult.
Daniel Sanchez, an assistant professor in MIT’s Department of Electrical Engineering and Computer Science, believes that it’s time to turn cache management over to software. This week, at the International Conference on Parallel Architectures and Compilation Techniques, Sanchez and his student Nathan Beckmann presented a new system, dubbed Jigsaw, that monitors the computations being performed by a multicore chip and manages cache memory accordingly.
In experiments simulating the execution of hundreds of applications on 16- and 64-core chips, Sanchez and Beckmann found that Jigsaw could speed up execution by an average of 18 percent — with more than twofold improvements in some cases — while actually reducing energy consumption by as much as 72 percent. And Sanchez believes that the performance improvements offered by Jigsaw should only increase as the number of cores does.
Location, location, location
In most multicore chips, each core has several small, private caches. But there’s also what’s known as a last-level cache, which is shared by all the cores. “That cache is on the order of 40 to 60 percent of the chip,” Sanchez says. “It is a significant fraction of the area because it’s so crucial to performance. If we didn’t have that cache, some applications would be an order of magnitude slower.”
Physically, the last-level cache is broken into separate memory banks and distributed across the chip; for any given core, accessing the nearest bank takes less time and consumes less energy than accessing those farther away. But because the last-level cache is shared by all the cores, most chips assign data to the banks randomly.
Jigsaw, by contrast, monitors which cores are accessing which data most frequently and, on the fly, calculates the most efficient assignment of data to cache banks. For instance, data being used exclusively by a single core is stored near that core, whereas data that all the cores are accessing with equal frequency is stored near the center of the chip, minimizing the average distance it has to travel.
Jigsaw also varies the amount of cache space allocated to each type of data, depending on how it’s accessed. Data that is reused frequently receives more space than data that is accessed infrequently or only once.
In principle, optimizing cache space allocations requires evaluating how the chip as a whole will perform given every possible allocation of cache space to all the computations being performed on all the cores. That calculation would be prohibitively time-consuming, but by ignoring some particularly convoluted scenarios that are extremely unlikely to arise in practice, Sanchez and Beckmann were able to develop an approximate optimization algorithm that runs efficiently even as the number of cores and the different types of data increases dramatically.
Quick study
Of course, since the optimization is based on Jigsaw’s observations of the chip’s activity, “it’s the optimal thing to do assuming that the programs will behave in the next 20 milliseconds the way they did in the last 20 milliseconds,” Sanchez says. “But there’s very strong experimental evidence that programs typically have stable phases of hundreds of milliseconds, or even seconds.”
Sanchez also points out that the new paper represents simply his group’s “first cut” at turning cache management over to software. Going forward, they will be investigating, among other things, the co-design of hardware and software to improve efficiency even further and the possibility of allowing programmers themselves to classify data according to their memory-access patterns, so that Jigsaw doesn’t have to rely entirely on observation to evaluate memory allocation.
“More and more of our computation is happening in data centers,” say Jason Mars, an assistant professor of computer science at the University of Michigan. “In the data-center space, it’s going to be very important to be able to have the microarchitecture partition and allocate resources on an application-by-application basis.”
“When you have multiple applications that are running inside a single box,” he explains, “there’s a point of interference where jobs can hurt the performance of each other. With current commodity hardware, there are a limited number of mechanisms we have to manage how jobs hurt each other.”
Mars cautions that a system like Jigsaw dispenses with a layer of abstraction between chip hardware and the software running on it. “Companies like Intel, once they expose the microarchitectural configurations through the software layer, they have to keep that interface over future generations of the processor,” Mars says. “So if Intel wanted to do something audacious with the microarchitecture to make a big change, they’ll have to keep that legacy support around, which can limit the design options they can explore.”
“However,” he adds, “the techniques in Jigsaw seem very practical, and I could see some variant of this hardware-software interface being adopted in future designs. It’s a pretty compelling approach, actually.”
MIT research shows that it may be time to let software, rather than hardware, manage the high-speed on-chip memory banks known as ‘caches.’
Publication Date:
Press Contact:

Credits:
Illustration: Christine Daniloff/MIT
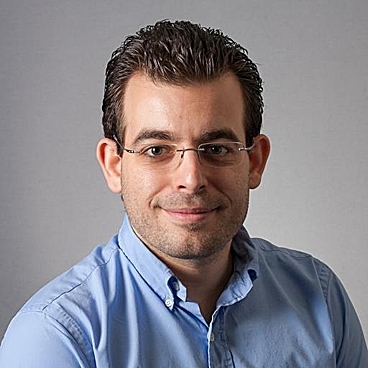
Caption:
Daniel Sanchez